Research scientists in Switzerland have developed and tested a robust AI model that automatically segments major anatomic structures in MRI images, independent of sequence, according to a study published in Radiology. In the study, the model outperformed other publicly available tools.
MRI provides detailed images of the human body and is essential for diagnosing various medical conditions, from neurological disorders to musculoskeletal injuries. For in-depth interpretation of MRI images, the organs, muscles and bones in the images are outlined or marked, which is known as segmenting.
“MRI images have traditionally been manually segmented, which is a time-consuming process that requires intensive effort by radiologists and is subject to inter-reader variability,” said Jakob Wasserthal, Ph.D., Radiology Department research scientist at University Hospital Basel in Basel, Switzerland.
“Automated systems can potentially reduce a radiologist’s workload, minimize human errors and provide more consistent and reproducible results.”
Dr. Wasserthal and colleagues built an open-source automated segmentation tool called the TotalSegmentator MRI based on nnU-Net, a self-configuring framework that has set new standards in medical image segmentation.
It adapts to any new dataset with minimal user intervention, automatically adjusting its architecture, preprocessing, and training strategies to optimize performance. A similar model for CT (TotalSegmentator CT) is being used by over 300,000 users worldwide to process over 100,000 CT images daily.
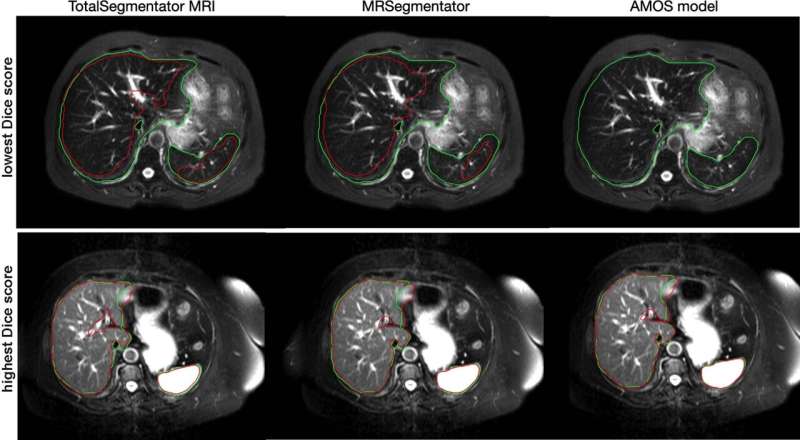
In the retrospective study, the researchers trained TotalSegmentator MRI to provide sequence-independent segmentations of major anatomic structures using a randomly sampled dataset of 616 MRI and 527 CT exams.
The training set included segmentations of 80 anatomic structures typically used for measuring volume, characterizing disease, surgical planning and opportunistic screening.
“Our innovation was creating a large data set,” Dr. Wasserthal said. “We used a lot more data and segmented many more organs, bones and muscles than has been previously done. Our model also works across different MRI scanners and image acquisition settings.”
To evaluate the model’s performance, Dice scores—which measure how similar two sets of data are—were calculated between predicted segmentations and radiologist reference standards for segmentations. The model performed well across the 80 structures with a Dice score of 0.839 on an internal MRI test set.
It also significantly outperformed two publicly available segmentation models (0.862 versus 0.838 and 0.560) and matched the performance of TotalSegmentator CT.
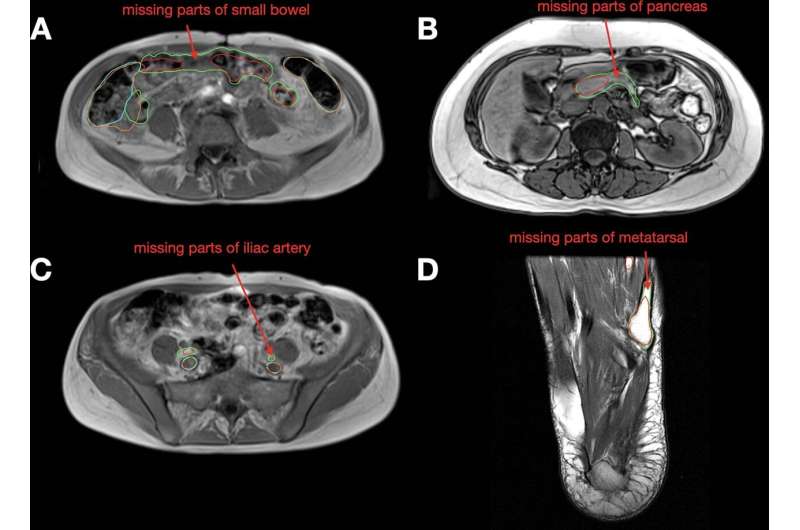
“To our knowledge, our model is the only one that can automatically segment the highest number of structures on MRIs of any sequence,” he said. “It’s a tool that helps improve radiologists’ work, makes measurements more precise and enables other measurements to be done that would have taken too much time to do manually.”
In addition to research and AI product development, Dr. Wasserthal said the model could potentially be used clinically for treatment planning, monitoring disease progression, and opportunistic screening.
More information:
TotalSegmentator MRI: Robust Sequence-independent Segmentation of Multiple Anatomic Structures in MRI, Radiology (2025).
Citation:
AI model automatically segments MRI images, reducing radiologist workload (2025, February 18)
retrieved 18 February 2025
from https://medicalxpress.com/news/2025-02-ai-automatically-segments-mri-images.html
This document is subject to copyright. Apart from any fair dealing for the purpose of private study or research, no
part may be reproduced without the written permission. The content is provided for information purposes only.