For the first time, researchers have used a surface normal nonlinear photodetector (SNPD) to improve the speed and energy efficiency of a diffractive optical neural network (ONN). The new device lays the groundwork for large-scale ONNs, which can perform high-speed processing at the speed of light in an extremely energy efficient manner.
Farshid Ashtiani from Nokia Bell Labs will present this research at Frontiers in Optics + Laser Science (FiO LS), which will be held 9–12 October 2023 at the Greater Tacoma Convention Center in Tacoma (Greater Seattle Area), Washington.
“Artificial intelligence (AI) is playing increasingly important roles in our lives. From large language models to autonomous cars, we see significant improvements enabled by AI,” explains Ashtiani.
“Neural networks, inspired by how the human brain learns and performs various tasks, are at the heart of the progress in AI. One of the widespread applications of neural networks is recognizing patterns and objects, which gives vision to machines. For instance, every autonomous car, or even semi-autonomous car, must understand its surroundings by being able to see things.”
“Conventionally, images are taken by cameras, converted to electrical signals, and processed using electronic processors such as CPUs or GPUs for object recognition,” said Mohamad Hossein Idjadi, Nokia Bell Labs.
“However, since the images are originally in optical domain (i.e., light), it can be faster and more energy efficient to process them optically using ONN. Among different technologies, ONNs based on spatial light modulators enable the optical processing of high-resolution images and videos. This processing requires nonlinear modules and camera sensors are conventionally used to introduce this needed nonlinearity, which takes several milliseconds. Our novel detector device makes this nonlinear processing 1000 times faster and more energy efficient than such cameras. This is crucial for the next generation of machine vision systems as we all need even faster intelligent gadgets that do not consume a lot of energy.”
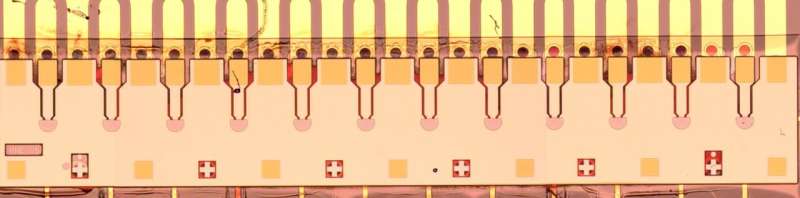
Free-space diffractive ONNs use spatial light modulators and are particularly promising for creating the large-scale networks of neurons necessary for image and video processing. However, the speed and energy efficiency of this type of ONN is usually limited by the image sensor used to implement the nonlinear activation function that implements multiple layers of neurons to create a deep neural network.
In the new work, the researchers proposed the use of an SNPD, that they had previously demonstrated as a high-speed electro-optic modulator, in high resolution diffractive ONNs. Testing showed that the SNPD had a 3-dB bandwidth of 61 kHz corresponding to less than 6 microseconds—about 1,000 times faster than the typical response time of camera sensors conventionally used in such ONNs. The sensor also consumes only about 10 nW/pixel, which is three orders of magnitude more efficient than a typical camera.
To test the new sensor in an ONN, the researchers input images into the convolution layer—the primary building block of the neural network. The convolution layer had 32 parallel 3 × 3 kernels with a stride of one and used the actually measured SNPD response as its activation function instead of the standard rectified linear activation function. With this simulation setup, the network achieved a test classification accuracy of about 97%, which is the same performance as using an ideal rectified linear activation function in the same network.
The research demonstrates the potential of using a SNPD in free-space diffractive ONNs. The fact that the detector is three orders of magnitude faster and more efficient than a camera makes it a promising candidate for use in large scale free-space ONN setups.
“We need to make a large number of our detector devices, potentially millions of them, to build a full vision system, and to compete with the high resolution provided by conventional cameras,” added Stefano Grillanda, Nokia Bell Labs. “The good news is that this is technologically possible. Another avenue to look into is to further reduce the footprint, energy consumption, and response time of the detector to make it an even better solution for future AI vision systems.”
Citation:
New detector paves the way to large-scale optical neural networks (2023, August 24)
retrieved 24 August 2023
from https://techxplore.com/news/2023-08-detector-paves-large-scale-optical-neural.html
This document is subject to copyright. Apart from any fair dealing for the purpose of private study or research, no
part may be reproduced without the written permission. The content is provided for information purposes only.