Through his research at Caltech, a local high school student revealed 1.5 million previously unknown objects in space, broadened the potential of a NASA mission, and published a single-author paper.
Matteo (Matthew) Paz’s article published in The Astronomical Journal describes a new AI algorithm he developed that led to these discoveries and that can be adapted by other astronomers and astrophysicists for their own research.
Paz has wanted to learn more about astronomy since his mother brought him to public Stargazing Lectures at Caltech when he was in grade school. In the summer of 2022, he came to campus to study astronomy and related computer science in the Caltech Planet Finder Academy led by Professor of Astronomy Andrew Howard.
Astronomer and IPAC senior scientist Davy Kirkpatrick served as Paz’s mentor.
“I’m so lucky to have met Davy,” Paz says. “I remember the first day I talked to him, I said that I was considering working on a paper to come out of this, which is a much larger goal than six weeks. He didn’t discourage me. He said, ‘OK, so let’s talk about that.’ He has allowed an unbridled learning experience. I think that’s why I’ve grown so much as a scientist.”
Kirkpatrick grew up in a farming community in Tennessee and realized his dream of becoming an astronomer with the help of his ninth-grade chemistry and physics teacher, Marilyn Morrison. She told him and his mother that he had potential and explained what courses he should take to prepare for college.
“I wanted to pass on that same sort of mentoring to someone else and hopefully many someone elses,” Kirkpatrick says. “If I see their potential, I want to make sure that they are reaching it. I’ll do whatever I can to help them out.”
Kirkpatrick also wanted to glean more insight from NEOWISE (Near-Earth Object Wide-field Infrared Survey Explorer), a now-retired infrared telescope that had scanned the entire sky in search of asteroids and other objects near Earth for more than 10 years.
While the NASA telescope was busy observing asteroids, it also detected the varying heat of other more distant, cosmic objects that flashed intensely, pulsated, or dimmed as they were eclipsed. Astronomers call these variable objects: hard-to-catch phenomena like quasars, exploding stars, and paired stars eclipsing each other.
But the data on these variable objects had not yet been harnessed. If the NEOWISE team could identify those objects and make them available to the astronomical community, the resulting catalog could provide insight into how the cosmic entities change over years.
“At that point, we were creeping up towards 200 billion rows in the table of every single detection that we had made over the course of over a decade,” Kirkpatrick says. “So my idea for the summer was to take a little piece of the sky and see if we could find some variable stars. Then we could highlight those to the astronomic community, saying, ‘Here’s some new stuff we discovered by hand; just imagine what the potential is in the dataset.'”
Paz had no intention of sifting through the data manually. His schoolwork had prepared him to bring a new viewpoint to the challenge. He’d taken an interest in AI during an elective that integrated coding, theoretical computer science, and formal mathematics.
Paz knew that AI trains best on vast, orderly datasets like the one Kirkpatrick had given him. And Paz had the advanced math knowledge that he needed to enjoy programming: He was already studying advanced undergraduate math in Pasadena Unified School District’s Math Academy, in which students finish AP calculus BC in eighth grade.
So Paz set off to develop a machine-learning technique to analyze the entire dataset and flag potential variable objects. In those six weeks, he began to draft the AI model, which began to show some promise. As he worked, he consulted with Kirkpatrick to learn the relevant astronomy and astrophysics.
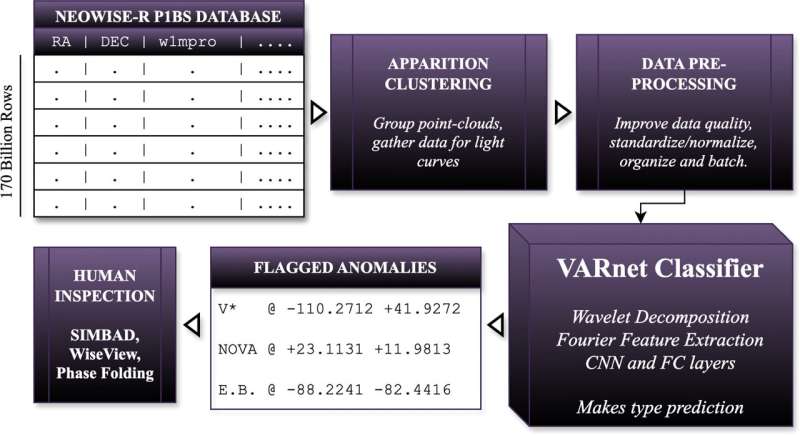
“Every meeting with Davy is 10% work and 90% us just chatting,” Paz says. “It’s been super cool just to have someone to talk to about science like that.”
Kirkpatrick also connected Paz with Caltech astronomers Shoubaneh Hemmati, Daniel Masters, Ashish Mahabal, and Matthew Graham, who shared their expertise in machine-learning techniques for astronomy and in the study of objects that vary on short and long timescales. Paz and Kirkpatrick learned that the particular rhythm of NEOWISE’s observations meant that it would be unable to systematically detect and classify many objects that either flashed once quickly or changed gradually over a long time.
As the summer concluded, there was still plenty to do. In 2024, Paz and Kirkpatrick again collaborated, and this time, Paz mentored other high school students.
Now, Paz has refined the AI model to process all of the raw data from NEOWISE’s observations and has analyzed the results. Trained to detect minute differences in the telescope’s infrared measurements, the algorithms flagged and classified 1.5 million potential new objects in the data. In 2025, Paz and Kirkpatrick plan to publish the complete catalog of objects that varied considerably in brightness in the NEOWISE data.
“The model I implemented can be used for other time domain studies in astronomy, and potentially anything else that comes in a temporal format,” Paz says. “I could see some relevance to (stock market) chart analysis, where the information similarly comes in a time series and periodic components can be critical. You could also study atmospheric effects such as pollution, where the periodic seasons and day-night cycles play huge roles.”
Now, while he finishes high school, Paz is a Caltech employee. He works for Kirkpatrick in IPAC, which manages, processes, archives, and analyzes data from NEOWISE and several other NASA and NSF–supported space missions. It’s Paz’s first paying job.
More information:
Matthew Paz, A Submillisecond Fourier and Wavelet-based Model to Extract Variable Candidates from the NEOWISE Single-exposure Database, The Astronomical Journal (2024). DOI: 10.3847/1538-3881/ad7fe6
Citation:
High school student uses AI to reveal 1.5 million previously unknown objects in space (2025, April 11)
retrieved 11 April 2025
from https://phys.org/news/2025-04-high-school-student-ai-reveal.html
This document is subject to copyright. Apart from any fair dealing for the purpose of private study or research, no
part may be reproduced without the written permission. The content is provided for information purposes only.